Machine Learning Product Manager Job Description: Key Responsibilities and Skills
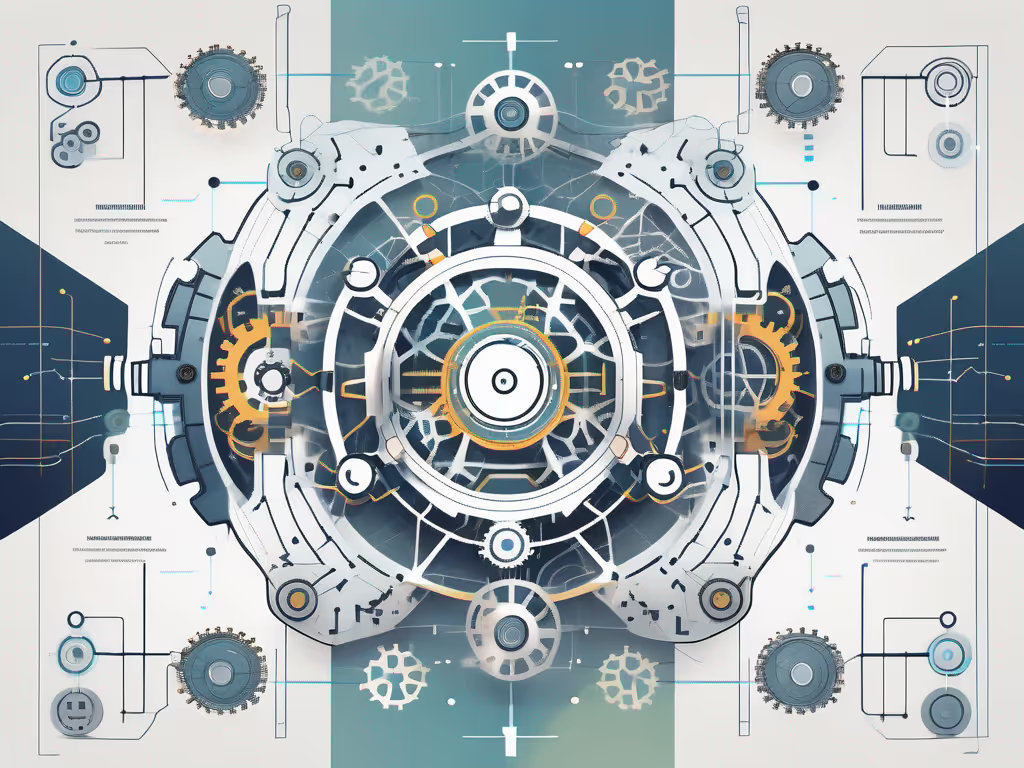
Understanding the Role of a Machine Learning Product Manager
The Machine Learning Product Manager (MLPM) plays a pivotal role at the intersection of technology, data science, and business strategy. As organizations increasingly leverage machine learning (ML) to enhance their offerings, the demand for skilled professionals to manage these products has surged. The MLPM is tasked with understanding complex technical concepts and translating them into strategically viable paths for product development.
In essence, an MLPM is responsible for guiding the development and deployment of machine learning products. They ensure these products align with business goals while also meeting user needs. This involves collaboration across various teams, including data scientists, engineers, and stakeholders, to create products that deliver value and drive growth. The MLPM must also stay abreast of the latest advancements in machine learning and artificial intelligence, ensuring that their products leverage cutting-edge technology to maintain a competitive edge in the market.
Key Responsibilities of a Machine Learning Product Manager
Machine Learning Product Managers are responsible for a range of crucial tasks that bridge technical and managerial duties. Key responsibilities include:
- Defining product vision and strategy, ensuring alignment with business objectives.
- Conducting market research to identify customer needs and industry trends.
- Collaborating with data science and engineering teams to develop and refine ML models.
- Setting product roadmaps and prioritizing features based on user feedback and business impact.
- Monitoring product performance and implementing improvements based on analytics.
- Stakeholder engagement, ensuring alignment and support for product initiatives.
These diverse responsibilities require a unique blend of technical knowledge, business acumen, and strong leadership skills. Additionally, MLPMs often need to navigate the ethical implications of machine learning applications, ensuring that products are developed responsibly and transparently. This includes addressing issues such as data privacy, algorithmic bias, and the broader societal impacts of deploying AI-driven solutions.
Skills Required for a Machine Learning Product Manager
Successfully navigating the role of an MLPM demands a versatile skill set. Here’s a breakdown of essential skills necessary for this position:
- Technical Understanding: Familiarity with machine learning algorithms, data architecture, and engineering principles.
- Analytical Skills: Ability to analyze data and derive insights to inform product decisions.
- Communication: Strong verbal and written communication skills to convey technical concepts to non-technical stakeholders.
- Project Management: Proficiency in managing projects, utilizing methodologies such as Agile or Scrum.
- Problem Solving: Critical thinking abilities to tackle complex challenges that arise during product development.
These skills enable MLPMs to effectively bridge the gaps between data science, engineering, and business strategies. Furthermore, adaptability is crucial in this rapidly evolving field, as MLPMs must be prepared to pivot their strategies based on new findings or shifts in market dynamics. Continuous learning and professional development are essential, as the landscape of machine learning and AI is constantly changing, requiring MLPMs to stay informed about emerging tools, techniques, and best practices.
The Importance of a Machine Learning Product Manager in a Tech Team
Having a dedicated Machine Learning Product Manager within a tech team is invaluable for several reasons. They serve as the crucial link connecting the technical and business sides of product development. Their deep understanding of both domains allows for more insightful decision-making, ensuring that products are not only innovative but also commercially viable.
Moreover, MLPMs foster an environment of collaboration among data scientists and engineers. By facilitating communication, they help prevent common pitfalls, such as misaligned priorities or misunderstandings about project goals. This harmony ultimately accelerates the product development cycle, delivering results to the market more efficiently. In addition, MLPMs often play a vital role in educating stakeholders about the capabilities and limitations of machine learning technologies, helping to set realistic expectations and drive informed decision-making throughout the organization. Their ability to articulate the value of ML initiatives can significantly enhance buy-in from leadership and ensure sustained investment in innovative projects.
Delving into the World of Machine Learning
Before diving deeper into the product management aspects, it is essential to understand the foundational elements of machine learning itself. As ML continues to evolve, it’s vital for product managers to have a grasp of the core concepts driving the technology.
Basic Concepts of Machine Learning
Machine learning is a subset of artificial intelligence that allows systems to learn from data, identify patterns, and make decisions with minimal human intervention. Here are some basic concepts that are fundamental to understanding ML:
- Supervised Learning: This technique uses labeled datasets to train algorithms, enabling them to make predictions based on new, unseen data.
- Unsupervised Learning: In contrast, this approach deals with unlabeled data, discovering hidden patterns without predefined outcomes.
- Reinforcement Learning: This method involves training models to make sequences of decisions by rewarding desired outcomes.
- Neural Networks: Inspired by the human brain, these networks consist of layers of interconnected nodes, useful for complex pattern recognition.
By grounding themselves in these concepts, Machine Learning Product Managers can more effectively manage projects and collaborate with technical teams. Understanding these principles not only helps in communicating with data scientists and engineers but also aids in making strategic decisions that align with the capabilities and limitations of machine learning technologies.
Furthermore, it is crucial to recognize the importance of data quality and quantity in machine learning. The performance of ML models is heavily dependent on the data they are trained on; thus, product managers must prioritize data collection and curation processes. Ensuring that the data is representative and free from bias can significantly enhance the model's accuracy and reliability, ultimately leading to better product outcomes.
The Impact of Machine Learning on Product Management
Machine learning profoundly affects product management, reshaping how products are developed, marketed, and iterated upon. With ML-driven insights, product managers can enhance customer experiences, personalize offerings, and make informed decisions based on data.
For instance, products that utilize ML can continuously adapt based on user interactions, leading to enhanced user satisfaction. Moreover, product managers can leverage analytics provided by ML systems to fine-tune marketing strategies and streamline operations. This dynamism represents a significant shift in product management, emphasizing the need for agility and responsiveness.
Additionally, the integration of machine learning into product management fosters a culture of experimentation. Product managers can implement A/B testing and other experimental methodologies to assess the effectiveness of different features or marketing approaches. By analyzing the outcomes of these experiments through the lens of machine learning, they can gain deeper insights into user behavior and preferences, allowing for more targeted and effective product enhancements. This iterative process not only improves product offerings but also strengthens the relationship between the product team and its users, creating a feedback loop that drives continuous improvement.
The Path to Becoming a Machine Learning Product Manager
For aspiring Machine Learning Product Managers, it is crucial to understand the educational and experiential prerequisites that equip individuals for the role. Various educational paths can lead to a successful career in this field.
Educational Background and Experience
Most Machine Learning Product Managers hold degrees in computer science, engineering, data science, or related fields. However, product management is an interdisciplinary field, and a background in business can also be beneficial. Understanding market dynamics, user experience, and business strategy can significantly enhance a product manager's ability to align machine learning solutions with customer needs and organizational goals.
Experience in software development, data analysis, or product management is highly valuable. Many successful MLPMs begin their careers in technical roles, which provide insights into the complexities of product development. These experiences allow them to build strong relationships with their technical teams, which is essential for effective product leadership. Moreover, familiarity with machine learning frameworks and tools, such as TensorFlow or PyTorch, can empower product managers to communicate more effectively with data scientists and engineers, ensuring that the product vision is both technically feasible and innovative.
Necessary Certifications and Training
In addition to formal education, pursuing relevant certifications can enhance marketability. Certifications in product management, agile methodologies, or data science are particularly beneficial. These credentials demonstrate a commitment to continuous learning and a solid understanding of the necessary concepts. Furthermore, certifications from reputable organizations can lend credibility, making candidates more attractive to potential employers.
Participating in workshops, online courses, and industry conferences can also provide valuable knowledge and networking opportunities. Engaging with the ML community can keep aspiring MLPMs at the forefront of evolving industry trends and practices. Networking with experienced professionals can lead to mentorship opportunities, providing insights into the nuances of the role and industry best practices. Additionally, contributing to open-source projects or collaborating on machine learning initiatives can not only bolster a resume but also foster a deeper understanding of the challenges and innovations within the field, ultimately shaping a more well-rounded product manager.
Navigating the Job Market for Machine Learning Product Managers
The job market for Machine Learning Product Managers is competitive yet dynamic, reflecting the growing importance of AI-driven technologies. Understanding the nuances of the job market can prepare candidates for successful job searches.
Understanding the Job Market
As businesses increasingly adopt machine learning technologies, the demand for skilled ML Product Managers continues to rise. Industries such as finance, healthcare, retail, and technology are particularly eager to integrate advanced machine learning capabilities into their operations. This trend is driven by the need for data-driven decision-making and the desire to enhance customer experiences through personalized services and predictive analytics.
However, candidates should be aware that the market can be selective. Companies often look for individuals who possess a blend of technical expertise and strong product management experience. This combination makes it essential for candidates to showcase their diverse skill sets and accomplishments effectively. Furthermore, understanding the specific challenges and opportunities within different sectors can provide candidates with a competitive edge, allowing them to tailor their applications to align with the strategic goals of potential employers.
Tips for Landing a Job as a Machine Learning Product Manager
To enhance chances of securing a position as an MLPM, consider the following strategies:
- Tailor Your Resume: Highlight relevant experiences and skills specific to machine learning and product management.
- Build a Portfolio: Create a portfolio showcasing successful projects that demonstrate your product management capabilities, particularly in ML domains.
- Network: Attend industry conferences, meetups, and engage with professionals in the field to build connections that can lead to job opportunities.
- Prepare for Technical Interviews: Brush up on ML concepts and be ready to discuss how these can impact product development during interviews.
- Stay Informed: Keep abreast of the latest trends in ML technology and industry developments to impress potential employers.
Additionally, consider seeking mentorship from experienced professionals in the field. A mentor can provide invaluable insights into the intricacies of the role and help you navigate the complexities of the job market. Engaging in online communities and forums dedicated to machine learning can also facilitate knowledge sharing and open doors to job leads. Moreover, pursuing relevant certifications in machine learning or product management can further bolster your qualifications, demonstrating a commitment to continuous learning and professional growth.
Finally, don’t underestimate the power of soft skills in this field. Effective communication, leadership, and problem-solving abilities are crucial for a Machine Learning Product Manager, as they often serve as the bridge between technical teams and stakeholders. Highlighting experiences where you successfully led cross-functional teams or managed complex projects can set you apart from other candidates and showcase your ability to drive results in a fast-paced, technology-driven environment.
Future Trends in Machine Learning Product Management
As machine learning continues to evolve, so too will the role of the Machine Learning Product Manager. Staying ahead of these trends will be crucial for professionals in the field.
The Evolution of the Machine Learning Product Manager Role
With the rapid pace of technological advancement, the expectations for Machine Learning Product Managers will likely grow. As more organizations embrace AI, MLPMs will need to develop a deeper understanding of ethics and governance in AI, ensuring that products adhere to legal standards and societal expectations.
Additionally, the role may increasingly focus on ensuring product sustainability and demonstrating how machine learning solutions deliver long-term benefits. This shift requires MLPMs to not only manage technical aspects but also consider broader implications, encouraging responsible innovation. They will need to engage with stakeholders across various departments to align product goals with ethical considerations, creating a holistic approach that integrates ethical AI practices into the product lifecycle.
Furthermore, as machine learning technologies become more complex, MLPMs will also need to enhance their communication skills. They will be tasked with translating intricate technical concepts into language that is accessible to non-technical stakeholders, ensuring that everyone involved—from engineers to executives—understands the product's value and implications. This ability to bridge the gap between technical and non-technical audiences will be essential for fostering collaboration and driving successful product outcomes.
Predicted Changes and Growth in the Field
The future of machine learning product management looks promising, with expected growth in job opportunities and advancements in technology. As machine learning becomes integrated into various sectors, the demand for professionals who can navigate its complexities will only escalate.
Moreover, the evolving landscape may give rise to new specializations within the role, such as focusing on AI ethics, user experience design, or advanced analytics. Product managers who embrace ongoing learning and adapt to these changes will be well-positioned to thrive in this dynamic field. The integration of interdisciplinary knowledge will become increasingly valuable, as MLPMs will need to collaborate with data scientists, UX designers, and legal experts to create well-rounded products that meet diverse needs.
In addition, the rise of no-code and low-code platforms is likely to democratize machine learning, allowing non-technical users to create and manage machine learning applications. This trend will shift some responsibilities from traditional MLPMs, requiring them to rethink their strategies and approaches to product management. They will need to focus on guiding users through these platforms, ensuring that the tools are user-friendly and that the resulting products maintain high standards of quality and ethical considerations. As the landscape of machine learning continues to evolve, the role of the Machine Learning Product Manager will be pivotal in shaping how these technologies are harnessed for innovation and societal benefit.
Additional Resources